Program Accomplishments
LDRD-funded research explores the frontiers of science and technology in emerging mission spaces, with projects guided by extremely creative, talented teams of scientists and engineers.
Featured Research
LDRD funded 258 projects in the fiscal year 2024. Here, we provide a closer look at a handful of projects that underscore the exciting, innovative research in this year’s LDRD portfolio. In addition, several PIs discuss their recent LRDR project experience in PI Perspectives.
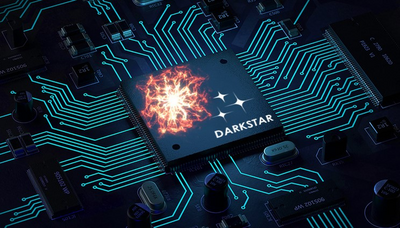
When materials are subjected to extreme environments, they face the risk of mixing together. This mixing may result in hydrodynamic instabilities, yielding undesirable side effects. Such instabilities present a grand challenge across multiple disciplines, especially in astrophysics, combustion and shaped charges — a device used to focus the energy of a detonating explosive, thereby creating a high velocity jet that is capable of penetrating deep into metal, concrete or other target materials.
To address the challenges in controlling these instabilities, researchers at Lawrence Livermore National Laboratory (LLNL) are coupling computing capabilities and manufacturing methods to rapidly develop and experimentally validate modifications to a shaped charge. This work, published in the Journal of Applied Physics, is a part of Project DarkStar — a Laboratory Directed Research and Development-funded strategic initiative aimed at controlling material deformation by investigating the scientific problems of complex hydrodynamics, shockwave physics and energetic materials.
“Like a hurricane, shock waves and the detonation of explosives are typically deemed ‘uncontrollable’ events. But we have made it our goal to control these complicated dynamical systems,” said DarkStar’s principal investigator Jon Belof.
The inspiration behind project DarkStar is deeply rooted in an unfinished line of research by Johnny von Neumann — a key member of the Manhattan Project and an expert in the nonlinear physics of hydrodynamics and shock waves. Having contributed to LLNL’s world-leading reputation in computing, von Neumann is often considered the most gifted mathematician of his time.
Applying modern technologies to von Neumann’s computational theories, the team employed artificial intelligence (AI) and machine learning (ML) to explore new, computationally optimized designs. The use of additive manufacturing — 3D printing — made it possible for researchers to rapidly realize even the most radical AI-designed components that would otherwise be considered “impossible” to create using traditional manufacturing methods.
To test their shaped charge designs — comprising a copper liner, a high explosive (HE) and a silicone buffer — the team conducted a total of 14 HE detonation experiments at LLNL’s High Explosives Applications Facility from 2022 to 2023. These experiments compared a baseline design, which did not utilize a buffer between the liner and the HE, against a design with an optimized buffer to demonstrate the effectiveness of the silicone buffer as an instability mitigation technique.
“Each of our designs went through optimization, manufacturing and detonation testing in less than three months,” said lead author Dylan Kline.
Once detonated, the metal liner is compressed and squeezed forward at about 5 kilometers per second, forming a high-velocity jet. The instability that this research aims to mitigate takes place when the explosive creates an impulse or “spike” at the materials interface, deforming and accelerating the metal (which has a high density) into the air around it (which has a low density). In this case, the instability or mixing of materials takes place when the jet forms in the air.
Kline said: “Our goal is to augment how this instability grows. If we can add something in our design to shape the shock waves, then we can control the way that energy is imparted on the metal liner.”
Flash X-ray radiographs taken during the detonation experiments reveal the silicone buffer’s ability to mitigate potential instabilities reliably and consistently.
Through their series of experiments, the team has uncovered several ground-breaking discoveries regarding hydrodynamic instabilities, including how to suppress an instability known as the Richtmyer–Meshkov Instability (RMI). RMI is of particular interest due to its unpredictable nature and role in materials undergoing extreme dynamic loading.
This research is directly applicable to aerospace engineering, as shaped charges are typically used for separating aircraft systems.
“This is just one case where having more powerful explosives and more effective ways of using them to manipulate metal could improve our industrial ecology,” Belof said.
Project DarkStar illuminates the potential of AI/ML to support a wide range of national security missions.
LDRD Project Title: Project DarkStar: Controlling Material Deformation
Principal Investigator:
Jon Belof
LDRD Project:
21-SI-006
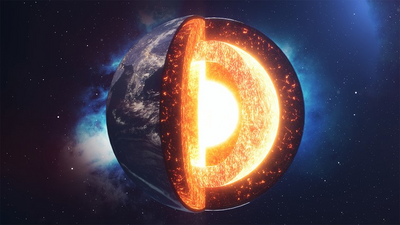
Researchers from Lawrence Livermore National Laboratory (LLNL) and Johns Hopkins University have unlocked new secrets about the interiors of super-Earth exoplanets, potentially revolutionizing our understanding of these distant worlds.
The focus of this work, magnesium oxide (MgO), a crucial component of Earth’s lower mantle, is believed to play a similar role in the mantles of massive rocky exoplanets. Known for its simple rock salt (B1) crystal structure and geophysical significance, MgO’s behavior under extreme conditions has long intrigued scientists.
Super-Earths, planets with masses and radii larger than Earth but smaller than ice giants like Neptune, are often inferred to have compositions similar to terrestrial planets in our solar system. Given the extreme pressures and temperatures within their mantles, MgO is expected to transform from its B1 structure to a cesium chloride (B2) structure. This transformation significantly alters MgO’s properties, including a dramatic decrease in viscosity, which can drastically affect the planet’s internal dynamics.
To pinpoint the pressure at which this transition occurs, the LLNL team and collaborators devised a novel experimental platform. This platform combines laser-shock compression with simultaneous measurements of pressure, crystal structure, temperature, microstructural texture and density — an unprecedented approach.
Conducting 12 experiments at the Omega-EP laser facility at the University of Rochester’s Laboratory for Laser Energetics, the scientists compressed MgO to ultra-high pressures of up to 634 GPa (6.34 million atmospheres) for several nanoseconds. Using a nanosecond X-ray source, they probed the atomic structure of MgO under these conditions. The results were striking: the B1 to B2 phase transition in MgO occurred within the 400-430 GPa pressure range at a scorching temperature of around 9,700 Kelvin. Beyond 470 GPa, B2-liquid coexistence was observed, with complete melting at 634 GPa.
“This study provides the first direct atomic-level and thermodynamic constraints of the pressure-temperature onset of the B1 to B2 phase transformation and represents the highest-temperature X-ray diffraction data ever recorded,” said LLNL scientist Ray Smith, author of a paper published in Science Advances. “These data are an essential for developing accurate models of super-Earth interior processes.”
The B1-B2 transition is a model for other structural phase transformations, attracting decades of theoretical research focused on the atomic pathways facilitating this change. Using a forward model to simulate X-ray diffraction conditions, the research team was able to clarify the mechanism of the B1-B2 transition in MgO.
“Our X-ray diffraction data provides direct measurements of atomic-level changes in MgO under shock compression and the first determination of a phase transition mechanism at deep mantle pressures of super-Earth exoplanets,” said LLNL scientist Saransh Soderlind.
LDRD Project Titles: Kinetics of Incipient Stages of Phase Transitions and Uncertainty Quantification and Experimental Design Using a Quantitative Forward Model for Kinematic X-Ray Diffraction
Principal Investigators:
Raymond Smith, and Joel Bernier
LDRD Projects:
15-ERD-012, 15-ERD-014, 17-ERD-014, and 20-ERD-044
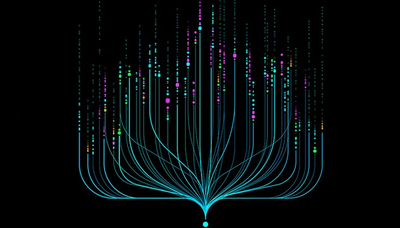
Amid the skyrocketing popularity of large language models (LLMs), researchers at Lawrence Livermore National Laboratory are taking a closer look at how these artificial intelligence (AI) systems perform under measurable scrutiny. LLMs are generative AI tools trained on massive amounts of data in order to produce a text-based response to a query. This technology has the potential to accelerate scientific research in numerous ways, from cyber security applications to autonomous experiments. But even if a billion-parameter model has been trained on trillions of data points, can we still rely on its answer?
Two Livermore co-authored papers examining LLM trustworthiness — how a model uses data and makes decisions — were accepted to the 2024 International Conference on Machine Learning, one of the world’s prominent AI/ML conferences.
“This technology has a lot of momentum, and we can make it better and safer,” said Bhavya Kailkhura, who co-wrote both papers.
More effective models
Training on vast amounts of data isn’t confirmation of a model’s trustworthiness. For instance, biased or private information could pollute a training dataset, or a model may be unable to detect erroneous information in the user’s query. And although LLMs have improved significantly as they have scaled up, smaller models can sometimes outperform larger ones. Ultimately, researchers are faced with the twin challenges of gauging trustworthiness and defining the standards for doing so.
In “TrustLLM: Trustworthiness in Large Language Models,” Kailkhura joined collaborators from universities and research organizations around the world to develop a comprehensive trustworthiness evaluation framework. They examined 16 mainstream LLMs — ChatGPT, Vicuna, and Llama2 among them — across eight dimensions of trustworthiness, using 30 public datasets as benchmarks on a range of simple to complex tasks.
Led by Lehigh University, the study is a deep dive into what makes a model trustworthy. The authors gathered assessment metrics from the already extensive scientific literature on LLMs, reviewing more than 600 papers published during the past five years.
“This was a large-scale effort,” Kailkhura said “You cannot solve these problems on your own.”
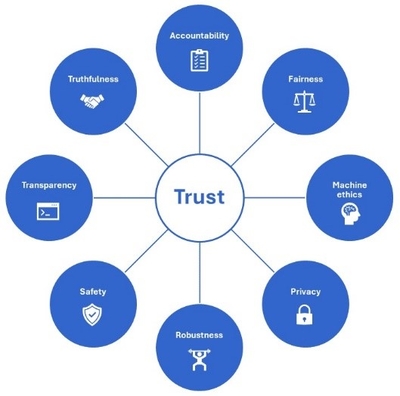
The team’s resulting TrustLLM framework defines the following dimensions. A fair model avoids discriminatory outcomes, such as refusing to respond to demographic stereotypes or gender biases. Machine ethics measures a model’s recognition of human morals and emotions, such as discerning between right and wrong if a user’s query implies harming another person. Privacy measures whether a model reveals sensitive information even if the training dataset contains, for example, phone numbers.
Determining LLM trustworthiness requires assessment of how a model performs against tasks representing different dimensions of trust. See the Task & Dataset section of the study’s website for a list of datasets and the dimension(s) they correspond to. For example, a dataset used to test privacy leakage consists of half a million emails from the Enron Corporation. Others contain product reviews or medical diagnoses for synthetic patient records.
Additionally, robustness refers to a model’s ability to handle anomalies or unexpected data, and safety refers to its resilience against data manipulation or exploitation attempts, such as a request to provide ingredients for an explosive device. A truthful model presents facts, states its limitations — such as if asked about a rapidly changing current event — and doesn’t “hallucinate” inaccurate or nonsensical information.
Two other dimensions are more difficult to measure because of the complex, large-scale nature of LLMs. Accountability means providing the origin(s) of the output, while transparency refers to detailed explanations of decision-making steps and rationale.
These standards are high. As recent copyright-related headlines point out, LLMs don’t cite their sources, nor do their owners assume responsibility for amalgamated datasets. Furthermore, training datasets can contain any number of imperfections, innocent or adversarial. A reasonably ethical model might be vulnerable to attacks.
“You can’t look at one single aspect of trustworthiness. You have to look at how the model performs in all the metrics,” Kailkhura said.
TrustLLM evaluations yielded mixed results. Most models refused to provide private information when instructed to follow a privacy policy, and answers to multiple-choice questions were more accurate than open-ended questions. Proprietary (closed-source) models tended to perform better than open-source models, which Kailkhura said could be attributed to companies’ investments in development. Still, the best performing model in identifying stereotypes achieved only 65% accuracy, and performance across models varied considerably when faced with unexpected data. The team also noticed a trend of over-alignment, where models’ safety scores are padded with false positives.
None of the tested models was truly trustworthy according to TrustLLM benchmarks. The good news, however, is that the study exposed where these models fail, which can encourage focus on trustworthiness as LLM developers continue to improve the technology.
“LLMs are foundational models of increasing importance to the Lab and its national security applications, which is why our AI safety research is critical,” Kailkhura said.
More efficient models
As LLMs scale up, computational performance will continue to pose a challenge. Another conference paper investigates trustworthiness in the context of compression, where a model is modified to reduce the amount of data and compute resources necessary for efficiency. For example, compressing a model from 13 billion to 7 billion parameters can cut its latency in half, depending on the computing hardware running it. State-of-the-art compression techniques are designed to boost a model’s response speed, but they often prioritize performance over trustworthy results.
“Our research provides practical guidance for producing lightweight, trustworthy LLMs in research projects or applications throughout the Lab,” said James Diffenderfer, who co-authored “Decoding Compressed Trust: Scrutinizing the Trustworthiness of Efficient LLMs Under Compression” alongside Kailkhura, Brian Bartoldson and colleagues from several universities. The team applied five compression techniques to leading LLMs, testing the effects on various trustworthiness metrics.
This work builds on prior research in convolutional neural networks (CNNs) with compression techniques like pruning (removing nonessential parameters from the model) and quantization (reducing the model’s computational precision) — both of which can be applied to LLMs alone or in combination.
“Past Livermore research with CNNs showed that these techniques could affect accuracy and robustness,” Diffenderfer said. “To make LLMs more ubiquitous and usable through compression, it’s important to perform these studies and identify strategies to make LLMs more efficient without degrading their trustworthiness.”
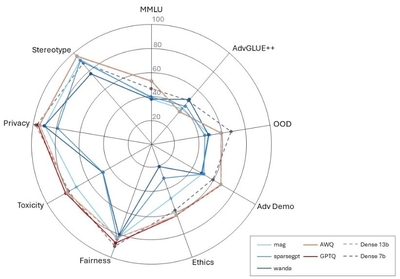
The team discovered that compression via quantization was generally better — i.e., the model scored higher on trust metrics — than compression via pruning. Furthermore, they saw improved performance of 4-bit quantized models on certain trustworthiness tasks compared to models with 3- and 8-bit compression. Even at the same compression level, some models scored higher on ethics and fairness tasks and lower on privacy tasks, for instance.
Comparison of compressed (blue and orange solid lines) and uncompressed (dashed lines) LLMs across nine trustworthiness benchmarks, where a higher score indicates better performance. For example, GPTQ scores high in the benchmarks on the lower left side of the graph, but is weaker in the benchmarks on the upper right side.
“The effect on performance for each task varied based on the quantization algorithm used to compress the LLM,” Diffenderfer said. “Certain forms of compression are better suited for deploying lightweight LLMs without overly compromising their trustworthiness.”
In some cases, compression can even improve a model’s trustworthiness. Yet too much compression can backfire, as trustworthiness scores dropped after a certain point.
“We wanted to find that line. How much can we compress these LLMs before they start behaving in a manner that is less useful?,” he said.
The rapid pace of LLM development raises new questions even as researchers answer existing ones. And with growing emphasis on this technology among the AI/ML community and at top conferences, understanding how LLMs work is the key to realizing their potential.
“By performing large-scale empirical studies, we observed certain compression algorithms improve the performance of LLMs while others harm the performance,” Diffenderfer said. “These results are valuable for producing efficient, trustworthy models in the future or designing improved architectures that are intrinsically more efficient and trustworthy.”
More valuable models
Livermore’s LLM research extends beyond these papers and reveals important insights into the high-stakes arena of AI safety, which is the focus of the October 2023 White House Executive Order. The Laboratory Directed Research and Development program funds projects that tackle different aspects of safety, and the Lab’s experts continually explore ways to maximize AI/ML benefits while minimizing risks. (Visit the Data Science Institute’s website for a list of high-profile publications on these topics.)
“Any major technological breakthrough results in both positive and negative impacts. In the Department of Energy and national security context, AI technologies come with the responsibility to be safe and secure,” Kailkhura said. “I have been working on this problem for a while now, and I am pretty confident that we will improve powerful AI models and solve key scientific challenges with them. We need to be proactive and move quickly.”
LDRD Project Title: Safe and Trustworthy ML
Principal Investigator:
Bhavya Kalikhura
LDRD Project:
20-ERD-014
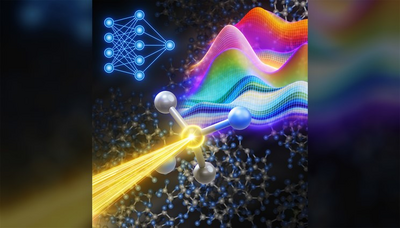
Lawrence Livermore National Laboratory (LLNL) scientists have developed a new approach that can rapidly predict the structure and chemical composition of heterogeneous materials.
In a new study in ACS Chemistry of Materials, LLNL scientists Wonseok Jeong and Tuan Anh Pham developed a new approach that combines machine learning with X-ray absorption spectroscopy (XANES) to elucidate the chemical speciation of amorphous carbon nitrides. The research offers profound new insights into the local atomic structure of the systems, and in a broader context, represents a critical step in establishing an automated framework for rapid characterization of heterogeneous materials with intricate structures.
Unraveling the atomic structure of heterogeneous materials, such as carbonaceous residues produced from the detonation of high explosives, has posed a significant challenge to materials scientists. The process is often labor-intensive, and in many cases, involves the use of empirical parameters.
To address this outstanding challenge, the team's integrated approach begins with the development of machine-learning potentials capable of efficiently exploring the vast configuration space of amorphous carbon nitrides as a representative system. This neural-network-based model enables the identification of representative local structures within the material, providing insights into how these structures evolve with chemical compositions and density.
By coupling these machine-learning potentials with high-fidelity atomistic simulations, the researchers establish correlations between local atomic structures and spectroscopic signatures. This correlation serves as the basis for interpreting experimental XANES data, allowing for the extraction of crucial chemical information from complex spectra.
“In our study, we aimed to tackle the longstanding challenge of characterizing detonation products and disordered materials in general by integrating computational methods with experimental techniques,” said Jeong, the first author of the paper.
“Our approach not only enhances our understanding of these materials but also lays the groundwork for similar studies across different material systems and characterization methods. For example, the approach can be readily employed to predict elemental speciation for a broad range of carbonaceous residues and provide inputs for improving detonation models,” said Pham, the principal investigator of the project.
The study’s findings represent a significant advancement in the field of materials science, offering a robust framework for elucidating the atomic speciation of disordered systems. Moreover, the versatility of the approach means it can be readily adapted to investigate other materials classes and experimental characterization probes, paving the way for real-time interpretation of spectroscopic measurements.
LDRD Project Title: Towards Automated Characterization of Heterogeneous Materials: A Case Study Using X-Ray Absorption Spectroscopy of Detonation Products
Principal Investigator:
Tuan Anh Pham
LDRD Project:
22-ERD-014
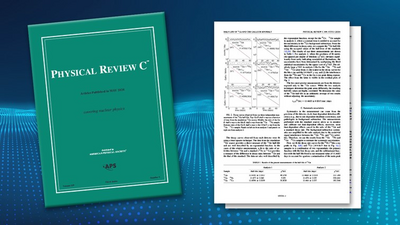
Searching for the elusive neutrino takes on many forms. Detectors consisting of many tons of gallium are used in several experiments because neutrino interactions can occur on the stable gallium-71 (71Ga) nucleus and transform it into a radioactive isotope of germanium (71Ge) with an 11-day half-life that can then be observed with traditional radiation detectors.
However, the rate of 71Ge production from these interactions has been observed to be short of expectations. This has emerged as what is referred to as “the gallium anomaly” — a significant discrepancy that occurs when electron neutrinos bombard gallium and produce the 71Ge.
This anomaly cannot be explained by current theories. As a result, it has given rise to speculation that it could be a signature that the neutrino can transform into other exotic particles, such as sterile neutrinos, which interact even less with matter than a normal neutrino; if confirmed, this would be a massive discovery.
Recently, a suggestion was made that this anomaly could instead be explained by something more mundane – a mismeasured half-life for the 71Ge nucleus. This is because the predicted rate of neutrino interactions depends on this half-life.
To test this possible explanation of the gallium anomaly, a team of scientists from Lawrence Berkeley and Lawrence Livermore national laboratories have determined the 71Ge half-life with a set of carefully performed measurements including two performed side-by-side with other long-lived radioactive isotopes with well-known half-lives. The research appears in Physical Review C.
The team was able to pin down the 71Ge half-life to a precision about four times better than any previous measurement. The work eliminates the mismeasurement of 71Ge as an explanation for the anomaly, which thus must have a different origin — possibly in the existence of a fourth neutrino type, called a sterile neutrino.
“The new half-life obtained by our team confirmed the earlier results, but put it on much firmer footing, definitively ruling out the possible explanation that the missing neutrinos were instead due to an incorrect 71Ge half-life,” said LLNL scientist and co-author Nick Scielzo. “Therefore, the gallium anomaly remains a true mystery – one that potentially still requires some kind of unexpected new neutrino behavior to understand.”
LDRD Project Title: The Biggest Bang for Your Buck: Pioneering a Nuclear-Science Program Guided by Artificial Intelligence
Principal Investigator:
Nicholas Scielzo
LDRD Project:
23-SI-004
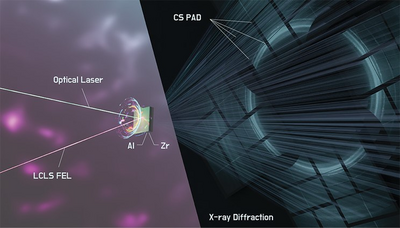
Shock experiments are widely used to understand the mechanical and electronic properties of matter under extreme conditions, like planetary impacts by meteorites. However, after the shock occurs, a clear description of the post-shock thermal state and its impacts on material properties is still lacking.
Lawrence Livermore National Laboratory (LLNL) scientists used ultra-fast X-ray probes to track the thermal response of aluminum and zirconium on shock release from experiments and found the resulting temperatures were much higher than expected. The research appears in the Journal of Applied Physics.
A shock wave is a large-amplitude mechanical wave across which pressure, density, particle velocity, temperature and other material properties change abruptly as the wave travels through the material. The shock compression process is thermodynamically irreversible, where a substantial portion of the energy in a shock wave goes into raising the entropy and temperature of the material.
The team used diffraction patterns from 100-femtosecond X-ray pulses to investigate the temperature evolution of laser-shocked aluminum-zirconium metal film composites at time delays ranging from 5 to 75 nanoseconds driven by a 120-picosecond short-pulse laser.
“We found significant heating of both the aluminum and zirconium after shock release, which can be attributed to heat generated by inelastic deformation,” said LLNL principal investigator Harry Radousky, a co-author of the study.
As it turns out, a conventional hydrodynamic model that uses typical descriptions of aluminum and zirconium mechanical strength and elevated strength responses (which might be attributed to an unknown strain rate) did not fully account for the measured temperature increase, which suggests that other strength-related mechanisms could play an important role in thermal responses under shock wave loading/unloading cycles.
“What we found is that a significant portion of total shock energy delivered by lasers become heat due to defect-facilitated plastic work, leaving less converted to kinetic energy,” said LLNL scientist Mike Armstrong, another co-author of the study. “This heating effect may be common in laser-shocked experiments but has not been well acknowledged. The high post-shock temperatures may induce phase transformation of materials during shock release.”
Armstrong said another potential application of the study is preserving magnetic records from planetary surfaces that have a shock history from frequent impact events.
Using the Matter in Extreme Conditions instrument at the Linac Coherent Light Source, the team found results showed much higher residual temperatures than expected from standard hydrodynamic release simulations, indicating there are other heat generating processes (void formation is one example) occurring during the release not usually included in these models.
LDRD Project Titles: Project DarkStar, Ultrafast Shock Kinetics of High-Z Materials with High Throughput
Principal Investigator:
Jon Belof
LDRD Projects:
21-SI-006 and 16-ERD-037
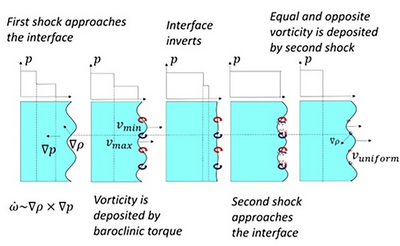
In a study published in the journal Physical Review Letters and noted as an Editor’s Suggestion, a team of physicists at LLNL have announced a significant scientific advance in controlling Richtmyer-Meshkov instability (RMI) in hydrodynamics. The team showed that specific sequences of shockwaves can effectively manage RMI, a phenomenon commonly observed in fluid dynamics when different densities of materials interact under shockwave conditions. Understanding RMI is highly important for laser-driven inertial confinement fusion (ICF) such as is pursued at the National Ignition Facility (NIF). RMI severely degrades ICF performance, so its mitigation may be viewed as an important steppingstone toward clean energy via fusion.
The study outlines two methods for generating these controlling shockwaves: a tailored drive approach and a material phase transition technique. The tailored drive method involves precise manipulation of shockwave intensity and timing, whereas the phase transition technique leverages changes in the material's state to induce the necessary shockwaves. In both scenarios, the underlying physical mechanism — discovered through extensive physics simulation work — is the same, relying upon the generation of vortices (regions of spinning or swirling material) after passage of the shockwave.
“Imagine part of a fluid that is spinning, like what happens if you pull the plug at the bottom of a bathtub. That vortex motion happening at a small scale is ultimately responsible for causing RMI and jetting when a shockwave breaks out of a material," said lead author of the study William Schill, staff scientist and deputy group leader of Material Dynamics and Kinetics in the Materials Science Division at LLNL. Schill elaborates further: “It turns out that, for very special conditions that we can create, it is possible to generate vortices that spin in the opposite direction, cancelling out the vortices generated by the shock and stabilizing the interface. In the case of a material undergoing phase transition from one state to another, the material may automatically suppress RMI, so to speak.”
To put the new theory to the test, an experimental campaign was conducted with team members at the Special Technology Laboratory (MSTS) in Santa Barbara, California, to generate the needed shockwaves under impact of a high-speed projectile. Firing a bullet at over 5,000 miles per hour and slamming it into a stationary piece of iron, a strong shockwave was generated that compressed the iron to a very high pressure. On the back of the sample were intentionally placed defects that are known to cause the hydrodynamic instability to form. Over a time period of several millionths of a second, x-ray images of the instability were taken and compared with theoretical predictions; remarkably, the instability was suppressed in agreement with the new vorticity cancellation theory. Mike Armstrong, deputy group leader in the Materials Science Division and the lead scientist for the experimental campaign, said: “We were surprised and excited by the results of this experimental campaign — the data from the shots showed very clearly that the method of controlling RMI worked exactly as predicted, demonstrating the practical feasibility of this approach in real-world scenarios.”
The control of Richtmyer-Meshkov instability has profound implications across multiple scientific fields, including inertial confinement fusion, astrophysics, materials science and energy research. This discovery may open new avenues for research in controlling high-velocity impacts and explosions, potentially leading to advancements in protective materials design and energy harnessing methods. The approach relied on use of advanced supercomputing resources and multiphysics simulation codes, highlighting the cutting-edge scientific capabilities provided to the U.S. by the Department of Energy laboratories.
LDRD Project Title: Project DarkStar
Principal Investigator:
Jon Belof
LDRD Project:
21-SI-006
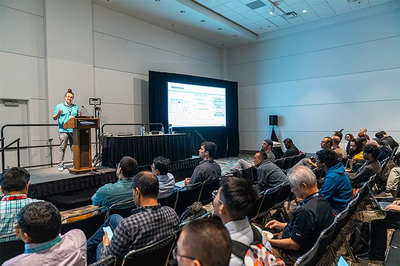
A Lawrence Livermore National Laboratory (LLNL)-led team has developed a method for optimizing application performance on largescale graphics processing unit (GPU) systems, providing a useful tool for developers running on GPU-based massively parallel and distributed machines.
A recent paper, which features four LLNL co-authors, describes a mechanism called Record-Replay (RR), which speeds up applications on GPUs by recording how a program runs on a GPU, and then replaying that recording to test different settings and finding the fastest way to run the program. The paper was a finalist for the Best Paper award at the 2023 International Conference for High Performance Computing, Networking, Storage and Analysis (SC23).
“We developed a tool that automatically picks up part of the application, moves it as an independent piece so you can start independently and then optimize it,” said lead author and LLNL computer scientist Konstantinos Parasyris. “Once it is optimized, you can plug it into the original application. By doing so you can reduce the execution time of the entire application and do science faster.”
In the paper, the authors describe how RR works and how it can be used to improve the performance of OpenMP GPU applications. Parasyris said the mechanism helps “autotune” large offload applications, thus overcoming a major bottleneck for speed in scientific applications.
As a case study, the authors demonstrated how RR was used to optimize performance of LULESH, a shockwave hydrodynamics code that simulates the behavior of materials under stress. By using the Record-Replay mechanism, researchers were able to optimize LULESH to run up to 50% faster on GPUs, an improvement that could make it possible to simulate much larger and more complex materials, key for many scientific and engineering applications.
“You can directly translate that as a 50% speed-up on an application,” Parasyris said. “Science is driven by how fast you can do observations, and in that case, we'll be able to increase the number of observations within a day; so, the [calculation] that previously took a day to do, would only take two-thirds of the day.”
By using the RR mechanism, researchers said they can test many different settings quickly and efficiently, making it possible to use Bayesian optimization — a method for finding the optimal settings for a program by testing different options and using statistics to determine which ones work best — on very large programs that would otherwise be too time-consuming to optimize.
“Every program has many parameters that you can use to optimize it, and those parameters can be combined if it's out there,” said LLNL scientist and co-author Giorgis Georgakoudis said. “If you find the best way to combine them, you can significantly reduce the execution time of the application, so that's our goal.”
Researchers said they plan to continue the work by investigating more use-cases facilitated by RR, such as tuning the compiler optimization pipeline, automatic benchmark generation and automated testing and debugging.
In addition to the Best Paper finalist, Parasyris and Georgakoudis also co-authored a paper presented at SC23 by LLNL intern Zane Fink on HPAC-Offload, a programming model allowing portable approximate computing (AC) to accelerate HPC applications on GPUs. The technique involves identifying and selectively approximating parts of the application that have low significance, resulting in significant performance improvements while minimizing quality loss.
The authors demonstrated the effectiveness of HPAC-Offload on several HPC benchmarks, conducting a comprehensive performance analysis of the tool across GPU-accelerated HPC applications. They found that AC techniques can significantly accelerate HPC applications (1.64x LULESH on AMD, 1.57x on NVIDIA GPUs) with minimal quality loss (0.1%). The team also provided insights into the interplay between approximate computing and GPU-based parallelism, which can guide the future development of AC algorithms and systems for these architectures. That paper also includes LLNL computer scientist Harshitha Menon as a co-author.
LDRD Project Title: Localizing and Explaining Performance Defects in Heterogeneous Applications
Principal Investigator:
Konstantinos Parasyris
LDRD Project:
23-ERD-022
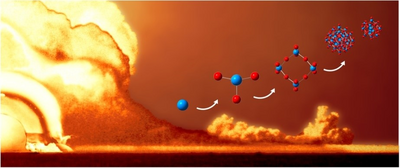
In the quest to understand how nuclear debris forms, a team of scientists at Lawrence Livermore National Laboratory (LLNL) has developed an approach to studying the oxidation mechanism of gas phase uranium in extreme environments.
In research recently published in Scientific Reports, the team outlined their work, which combined experimental data from a plasma flow reactor — a unique experimental platform built at LLNL — with advanced computational techniques to shed light on the intricate mechanics of gas phase uranium oxidation at atmospheric pressure.
By employing a Monte Carlo genetic algorithm, a hybrid model that uses random sampling and natural selection, researchers optimized a reaction mechanism based on optical emission spectra measurements from the plasma flow reactor, explained Mikhail Finko, lead researcher on the project. Using this method, reaction rates of gaseous uranium oxidation were then backed out from experimental data instead of being calculated from first principles.
“The methodology we employed not only identifies dominant reaction pathways and rates but also paves the way towards producing a comprehensive, experimentally validated reaction mechanism, a crucial element for modeling nuclear debris formation,” Finko said.
The algorithm LLNL researchers used identified four dominant reaction pathways for forming diatomic uranium oxides and determined the corresponding reaction rates. Furthermore, due to the presence of water in the system, the optimization identified the hydroxyl (OH) radical, a highly reactive chemical species composed of oxygen and hydrogen, as a dominant molecule for producing uranium oxides.
In part due to the development of convenient experimental systems for studying gas phase uranium chemistry, the subject has seen increased interest in recent years. While advances in qualitative understanding of gaseous uranium oxidation mechanics have been made, obtaining validated quantitative reaction rates has remained a challenge, Finko said. The results of this research are directly relevant for the field of material chemistry in extreme environments, as existing reaction mechanisms are not yet well validated.
LDRD Project Title: Identifying the Influence of Environmental Effects on Post-Detonation Chemistry and Debris Formation
Principal Investigator:
Kimberly Knight
LDRD Project:
20-SI-006
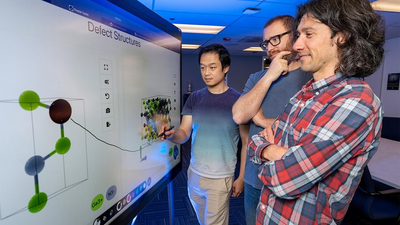
Point defects (e.g. missing, extra or swapped atoms) in crystalline materials often determine the actual electronic and optical response of a given material. For example, controlled substitutions in semiconductors like silicon are the backbone of modern technology. Despite their importance, point defects are notoriously difficult to simulate and characterize, particularly across wide regions of the periodic table.
Researchers at Lawrence Livermore National Laboratory (LLNL) have now created software as part of its open-source software distribution that can efficiently and effectively automate and analyze these types of calculations.
The authors demonstrated the fully automated approach on several technologically important materials. including gallium nitride (the basis of all modern solid-state lighting), gallium oxide (an emerging ultrawideband gap semiconductor) and strontium titanate (a widely studied common mineral), with the work recently published in the Journal of Applied Physics and selected as an Editor’s Pick as part of a special issue on “Defects in Semiconductors.”
“This work has enabled us to look more systematically at different types of defects in materials that exhibit the behavior we have been looking for,” said Lars Voss, a co-author of the work.
“We have been doing these types of calculations by hand for years, but modern advances in high-throughput computing and database software have made this a more practical and flexible approach,” said LLNL scientist Joel Varley, also a contributing author on the paper.
The study and open-source software developed as part of the project has attracted interest from a number of international research teams and industry, the researchers said.
“Now that we have developed a framework to streamline this approach with modern databasing practices, this opens up a straightforward path to curate data for machine-learning approaches to be systematically applied to point-defect properties by the community,” said Jimmy Shen, lead author on the paper.
LDRD Project Title: Ultrawide Bandgap Laser Addressable Photoconductors
Principal Investigator:
Lars Voss
LDRD Project:
22-SI-003
Scientific Leadership and Service
LDRD projects are distinguished by their mission-driven creativity. LDRD-funded research often launches stellar careers, initiates strategic collaborations, produces game-changing technical capabilities, and even lays the foundation for entirely new fields of science. It is no surprise that every year, LDRD principal investigators from LLNL are recognized for the groundbreaking results of a project or long-term contributions to their fields. The following examples highlight recognition received during fiscal year 2024, attesting to the exceptional talents of these researchers and underscoring the vitality of Livermore’s LDRD Program.
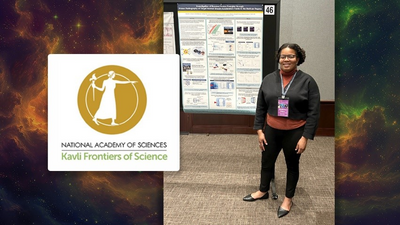
LLNL’s Raspberry Simpson named Kavli Fellow
Raspberry Simpson, a Lawrence Fellow in Lawrence Livermore National Laboratory’s (LLNL) National Ignition Facility and Photon Science (NIF&PS) Directorate, has been named a National Academy of Sciences (NAS) Kavli Fellow.
As a new Kavli fellow, Simpson participated recently in the annual NAS Kavli Frontiers of Science symposium in Irvine, California. NAS invited outstanding young scientists to discuss advances and opportunities in a wide variety of disciplines, including astrophysics, space science, space technology and xenobots — synthetic lifeforms made from components of living cells designed to perform robotic functions.
“It was definitely an honor to be invited to attend this meeting and to be able to meet other early-career scientists in other fields and learn about the NAS, which has the mission to inform the government on a wide array of scientific topics,” Simpson said. “I was able to make important connections with scientists outside of my field, such as individuals from Lawrence Berkeley National Laboratory that work in astrophysics and people from NASA Goddard."
The Goddard Space Flight Center is the National Aeronautics and Space Administration’s premiere space flight complex in Greenbelt, Maryland.
“I’m happy to be included in the NAS and Kavli communities and hope to be able to contribute in the future on their reports on high-energy-density (HED) science, high-brightness sources or fusion energy,” she said.
Simpson presented a poster on her postdoctoral fellowship titled “Investigation of Boosted Proton Energies in the Multi-ps Regime for Applications to Proton Fast Ignition.”
“It was a nice experience to be able to practice my own scientific communication and explain my work to individuals who are not necessarily in my field,” she said.
Simpson joined LLNL in 2022. She works on developing new experimental and machine-learning tools to optimize laser-driven secondary particle sources. Her work aims to address the need for next-generation diagnostics, machine-learning methods and analysis tools to be able to perform laser experiments at faster rates.
Simpson received her Ph.D. in nuclear engineering with a focus on plasma physics from the Massachusetts Institute of Technology. She completed most of her Ph.D. research at LLNL under the direction of physicist Tammy Ma, focusing on the investigation of laser-driven particle acceleration for the development of tunable ion sources for applications in HED science.
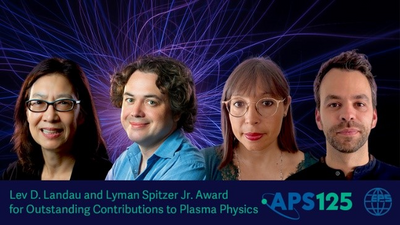
Two LLNL physicists honored for international collaboration
Lawrence Livermore National Laboratory (LLNL) physicists Hye-Sook Park and George Swadling, along with Anna Grassi of France’s Sorbonne University and former Lawrence Fellow Frederico Fiuza of Portugal’s Técnico Lisboa, received the 2024 Lev D. Landau and Lyman Spitzer Jr. Award for Outstanding Contributions to Plasma Physics. The award is jointly sponsored by the Plasma Physics Divisions of the American Physical Society and the European Physical Society.
The team was cited for “critical advancement in the understanding of the particle acceleration physics in astrophysically relevant shocks through theoretical analysis and experiments at the National Ignition Facility.”
“It is an honor to receive this award,” Park said. “We have been studying collision-less shocks in the laboratory using high-power lasers such as NIF and Omega by creating similar conditions in dimensionless units. We were particularly interested in how cosmic ray particles are accelerated to very high energy.”
Many theories exist, but observing the exact mechanisms is difficult.
“The physics of collision-less shocks is fascinating,” Swadling said. “They are common in astrophysics, forming, for example, at the interface between expanding supernova explosions and the interstellar medium. Only with the laser power and energy available at NIF are we able to design experiments at the spatial and temporal scales required to study their formation in the laboratory.”
This work showed that the electrons in the high-velocity plasma gain enough energy through the interplay of self-generated magnetic fields and the shock front, and these electrons are injected into another acceleration mechanism called a first-order Fermi process. The novel diagnostics on NIF measuring particle acceleration and the plasma conditions with novel simulations enabled these findings.
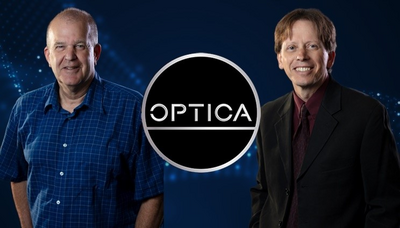
Two LLNL researchers named to Optica’s 2024 class of senior members
Lawrence Livermore National Laboratory (LLNL) researchers Paul Armstrong and Brent Stuart have been named senior members of Optica. The professional society’s senior membership status recognizes members with more than 10 years of professional experience in optics or an optics-related field.
The 2024 class joins a distinguished group of scientists, engineers, entrepreneurs and innovators who have demonstrated exemplary professional accomplishments in optics and photonics.
Paul Armstrong
Armstrong, an engineer in the National Ignition Facility and Photon Science Directorate, manages an effects laboratory in the Department of Defense Technologies program and investigates challenging problems in material science and laser-matter interaction.
"I feel fortunate to have spent my entire career at LLNL tackling difficult problems of national importance, supported by one of the best workforces around,” he said. “When you combine that with the opportunities afforded by Optica, amazing things happen. I am honored to have been selected as a senior member of such a respected professional society.”
Armstrong earned his bachelor's degree in Laser Optical Engineering Technology from the Oregon Institute of Technology and immediately joined LLNL. He began his career performing research in femtosecond lasers and their applications, including hardware-centric evolutionary algorithms and advanced control systems. He subsequently shifted focus to developing early concepts in inertial fusion energy drivers and other solid-state laser front-ends, such as the L3 HAPLS (High-Repetition-Rate Advanced Petawatt Laser System) now installed at ELI-Beamlines in the Czech Republic. Over his 30 years of work in photonics-related research, he has developed several novel instruments and processes, garnering multiple patents and three R&D100 awards. His current research concentrates on infrared imaging, spectroscopy and thermography.
Brent Stuart
Stuart, a staff scientist in the Physical and Life Sciences Directorate, is the operations manager for the Jupiter Laser Facility (JLF). He oversees all aspects of laser development and innovation at JLF. Recently, he led a large facility modernization effort for the facility, which resulted in multi-million-dollar investments by LLNL and the DOE Office of Science to redesign and rebuild the laser beamlines, power conditioning systems and diagnostics. The laser reopened for user experiments in 2023.
"I’m honored by this recognition, and thankful for all the exciting opportunities and amazing colleagues here at LLNL that made this award possible,” Stuart said.
He earned his bachelor’s degree in physics from Caltech and his Ph.D. in engineering/applied science from the University of California, Davis. After demonstrating a new ultraviolet laser based on the sulfur monoxide molecule for his thesis work, Stuart joined LLNL to work on the Nova petawatt laser, where he built up the front-end of the system and performed seminal investigations into the mechanisms of sub-picosecond laser ablation and materials processing applications.
Stuart also has delivered femtosecond machining systems to the Y-12 Plant and LLNL’s High Explosives Applications Facility; the trigger laser for the Dynamic Transmission Electron Microscope; and novel active remote-sensing systems. His leadership in laser design and operations led to many applications in particle acceleration, high-field laser-matter interactions, materials characterization, remote sensing and materials processing. Stuart has served on many major Optica conference program committees such as CLEO, Photonics West and Frontiers in Optics.
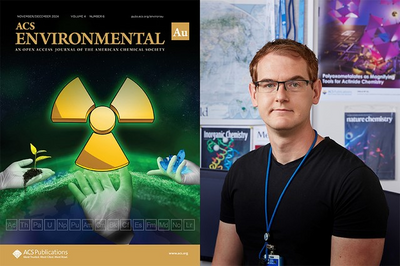
LLNL’s Gauthier Deblonde selected as ‘Rising Star’
Lawrence Livermore National Laboratory (LLNL) staff scientist Gauthier Deblonde has been named a 2024 “Rising Star“ by the American Chemical Society for his work in environmental science.
Deblonde’s research as well as this year’s cohort of winners will be featured in a special issue of the American Chemical Society journal ACS Environmental Au. Deblonde’s research also has been selected for the front cover of the journal’s current issue.
As a staff scientist in LLNL’s Nuclear and Chemical Sciences Division in the Physical and Life Sciences Directorate, Deblonde’s work focuses on developing new techniques to decipher the chemistry of radioactive elements.
Deblonde has always been fascinated by “difficult-to-study” materials. So, he naturally turned to nuclear sciences, and particularly the chemistry of heavy elements and “actinides” (a family of 15 radioactive elements, which includes uranium and plutonium).
Most of these elements only exist in trace amounts in nature or need to be produced in nuclear reactors and, as a result, we know very little about their chemical properties – relative to other non-radioactive materials. Deblonde’s research focuses on developing new strategies to probe the chemistry of these rare, toxic and elusive chemical elements.
"Most people fear radioactive materials, but a lot of this is irrational and not based on facts,” he said. “That’s why it is important to study such materials — from fundamental research to applied sciences — so that we better understand their interactions with the environment, prevent potential hazards and still take full advantage of their truly unique properties for national security and civilian applications."
Current studies in his lab focus on the interactions between metal ions and chelators (natural small molecules, polyoxometalates, synthetic ligands or macromolecules, like proteins). Such studies have broad implications, ranging from separation technologies and medical applications to nuclear sciences, strategic metal mining and the environmental behavior of heavy metals.
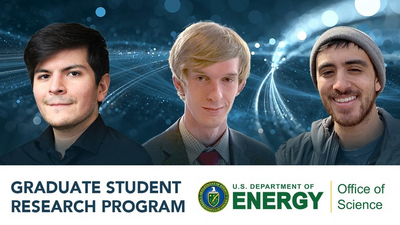
Three selected as Graduate Student Research program recipients
Three graduate students have earned Department of Energy (DOE) Office of Science Graduate Student Research (SCGSR) Program awards to perform their doctoral dissertation research at Lawrence Livermore National Laboratory (LLNL). The prestigious award helps cover living expenses and travel for 60 students from universities across the nation. Their proposed research projects address scientific challenges central to Office of Science mission areas from nuclear physics to environmental systems and advanced manufacturing.
“The Graduate Student Research program is a unique opportunity for graduate students to complete their Ph.D. training with teams of world-class experts aiming to answer some of the most challenging problems in fundamental science,” said Harriet Kung, acting director of the DOE Office of Science. “Gaining access to cutting-edge tools for scientific discovery at DOE national laboratories will be instrumental in preparing the next generation of scientific leaders.”
Andrew Marino from the Colorado School of Mines, Ricardo Monge Neria from Case Western Reserve University and Anthony Stewart from the University of Washington will arrive at the Lab this summer to start their fellowships.
Through world-class training and access to state-of-the-art facilities and resources at DOE national laboratories, SCGSR prepares graduate students to enter jobs of critical importance to the DOE mission and secures the national position at the forefront of discovery and innovation.
Andrew Marino
Under the direction of staff scientist Stephan Friedrich, Marino will continue to contribute to a sterile neutrino project with superconducting radiation detectors (nicknamed the “BeEST”), that he started working on while taking classes at Colorado School of Mines.
"I'm super excited to have received the SCGSR award and to have the opportunity to work here to further my thesis research with Stephan,” Marino said. “I've been mostly working with the data taken with our detectors by someone else and am really looking forward to taking some data myself."
In school, Marino worked with Superconducting Tunnel Junction (STJ) radiation detectors to measure nuclear recoils. While at LLNL, he will work on the method of STJ detector calibration. In the past, the team has used a laser to compare the energy of laser photons (easily measured) to that of the nuclear recoils (much harder).
“However, nobody has confirmed yet that there's no weird offset associated with low-energy events [missing some photons, for instance] or the difference between photons and charged particles [i.e. nuclei],” Marino said. “I'm hoping that I'll get some great data from STJs and confirmation that our calibration methodology is working accurately.”
Ricardo Monge Neria
As a physics student specifically studying techniques to optimize chemical separations, Monge Neria will work under the direction of staff scientist Dan Park on rare-earth element (REE) research. He is an experimental biophysics Ph.D. candidate on paper, but his work has mostly focused on studying chemical separations through the lens of single-molecule fluorescence microscopy. He studies the materials science aspect of liquid chromatography-based chemical separations, studying different porous and functionalized materials for difficult separations like rare earths and chiral chemicals.
Monge Neria will work with Park’s team to study protein-based approaches for the separation of crucial rare-earth metals, including measurements for an application to remove metals in waste streams.
"This is a great chance to simultaneously broaden my research skills into more biological and physical chemistry techniques, as well as expand upon my thesis work,” Monge Neria said. “In my mind, I like to view this opportunity as a ’mini post-doc’ experience where I'll get to work more in-depth in a related research topic. And I hope to make new connections with other researchers in the field, as well as experience the unique national laboratory environment as I head into the next steps of my career."
Anthony Stewart
Forest ecology and soil sciences Ph.D. candidate Anthony Stewart will work under the direction of staff scientist Katerina Georgiou on soil-carbon science and persistence. Soil organic matter stores more carbon than both the atmosphere and vegetation combined, and soils in the Pacific Northwest — where Stewart conducts fieldwork — are particularly rich in carbon, in part due to the presence of forested wetlands. Importantly, this carbon also can be hundreds to thousands of years old, but it is still uncertain in what forms this soil carbon is stored and how stable it is in hydrologic gradients in these landscapes. Stewart’s field of study is soil science; more specifically, soil carbon and how it persists in the soil and is distributed across landscapes.
"I’m grateful for the opportunity to work at such a prestigious institution with a great mentor and collaborators toward addressing key soil science challenges,” Stewart said. “I’m hoping to directly assess how long soil carbon persists in the soil samples I collected. Additionally, I’m planning to measure geochemical properties to explore some of the mechanisms of carbon stabilization."
“We are really excited that Anthony was awarded this fellowship and will join us at the Lab,” Georgiou said. “His SCGSR project will focus on the controls of soil-carbon science and persistence across a wetland-upland gradient in the Pacific Northwest. It is an ambitious project that will leverage key expertise and facilities at the Lab and will also complement our research program in the Nuclear and Chemical Science division.”
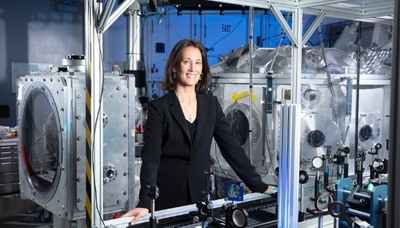
Félicie Albert elected vice chair of APS Division of Plasma Physics
Established in 1959, the objective of DPP is the advancement and dissemination of the knowledge, understanding, and applications of plasmas — assemblages of charged particles of natural and laboratory origin.
In this four-year leadership commitment, Albert will serve as chair of the APS-DPP fellowship committee in the first year and then chair the program committee for the APS-DPP annual meeting in the second. In her third year of service, Albert will chair the division, where she will lead the APS-DPP executive committee in running the division, proposing new initiatives and working with APS to ensure a vibrant and thriving plasma physics community. In her fourth year, she will serve as past chair of the division.
Albert first became a member of the APS and attended the meeting of the APS-DPP as an LLNL postdoctoral researcher in 2009.
"There has never been a better time to be part of DPP,” said Albert. “Scientifically, we’ve seen so many breakthroughs in our field and are seeing the prospects of a clean energy source provided by fusion, both from magnetic and inertial confinement, become a reality. And plasmas are so much more. They have unparalleled breadth in temperature, density, and pressures, beat particle acceleration energy-records, help us understand space and planets, devise new means of propulsion, and have the potential to revolutionize medical and industrial applications."
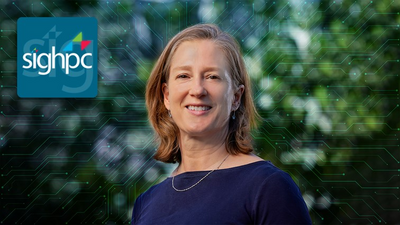
LLNL’s Kathryn Mohror honored with prestigious technical computing award
The Association for Computing Machinery’s (ACM) Special Interest Group on High Performance Computing (SIGHPC) on Sept. 3 announced it has awarded Lawrence Livermore National Laboratory’s (LLNL) Kathryn Mohror with its prestigious Emerging Woman Leader in Technical Computing (EWLTC) Award.
Mohror, a Distinguished Member of Technical Staff at LLNL and deputy director of the Laboratory Directed Research and Development program, is a leading HPC researcher with a focus on input/output (I/O) and programming models and tools designed for exascale computing. The award recognizes her remarkable achievements in high-performance computing (HPC) and her dedication to advancing the HPC community through her leadership, service and mentorship, according to SIGHPC.
"I am truly honored and excited to receive the Emerging Woman Leader in Technical Computing Award from SIGHPC,” Mohror said. “This recognition by my peers and the broader HPC community is incredibly meaningful to me. The award reflects the importance of collaboration, mentorship and innovation in driving our field forward. I am deeply grateful to be part of a community that values not just technical excellence, but also service, leadership and the positive impact we can have together. I am inspired to continue pushing the boundaries of what’s possible in HPC and to support the next generation of leaders in our field."
A computer scientist in the Parallel Systems Group within the Center for Applied Scientific Computing (CASC) at LLNL, Mohror is widely recognized for her work on HPC input/output (I/O) performance and parallel programming. Her research focuses on developing scalable solutions for extreme-scale computing systems, enhancing their performance, reliability and usability.
“We are incredibly proud of Kathryn and excited that she has received this well-deserved recognition,” said CASC Director Jeffrey Hittinger. “This award is a testament to her exceptional technical expertise, innovative research, and commitment to advancing the field of high performance computing. Kathryn’s work on scalable I/O systems and checkpointing libraries has greatly enhanced our ability to leverage HPC resources for critical national security missions and scientific discoveries. Beyond her technical achievements, Kathryn has been a remarkable leader and mentor both within the Lab and the broader HPC community. Her dedication to excellence, collaboration and service has had a profound impact, and we are fortunate to have her as a member of CASC.”
Mohror earned her Ph.D. in computer science in 2010, her master’s degree in computer science in 2004, and her bachelor’s degree in chemistry in 1999, all from Portland State University. Her innovative research has earned her several honors, including the 2022 Oppenheimer Science and Energy Leadership Program Fellowship and the 2019 DOE Early Career Research Award.
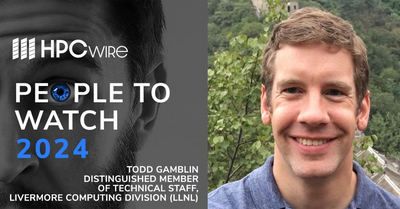
LLNL’s Gamblin named to HPCwire’s 'People to Watch' list for 2024
The high performance computing (HPC) publication HPCwire has selected Lawrence Livermore National Laboratory computer scientist Todd Gamblin as one of its “People to Watch” in HPC for 2024.
A distinguished member of technical staff in Livermore Computing, Gamblin is best known in the HPC community for creating Spack, a popular open source HPC package management tool that won an R&D 100 Award in 2019. Spack has grown to involve more than 1,300 contributors from more than 300 organizations and was the official deployment tool for the Department of Energy’s Exascale Computing Project. Spack has also become the package manager of choice for supercomputers around the world.
In addition to leading the Spack project, Gamblin is a co-founder of the High Performance Software Foundation (HPSF), a Linux Foundation project umbrella currently in the formation stage. The foundation is expected to launch in May, and seeks to build, promote and advance a portable software stack for HPC by supporting and encouraging adoption of key HPC open source software projects.
"I am honored and humbled to be recognized by HPCwire as one of their People to Watch for 2024,” Gamblin said. “This honor is a testament to the collective efforts of myself and my team, collaborators on HPSF, Livermore Computing and the entire Spack community. I am proud to be featured among the luminaries on this list and acknowledged alongside them as champions for innovation in the field of HPC."

Inaugural National Lab Research SLAM showcases early-career researchers
Gathered in the Congressional Auditorium on Wednesday, Nov. 15, 2023, 17 early-career researchers used three minutes and a single slide to present their pioneering research during the inaugural National Lab Research SLAM. Representing each of the 17 Department of Energy (DOE) national laboratories, finalists presented in four research categories: Energy Security, National Security, Environmental Resilience and Scientific Discovery. Sponsored by the House Science and National Labs Caucus and the Senate National Labs Caucus, the first-of-its-kind event heightened competition and collaboration while raising visibility of the national laboratory system and federal research priorities. The winners were: Scientific Discovery: Theresa Kucinski, Los Alamos National Laboratory; National Security: Brandon Zimmerman, Lawrence Livermore National Laboratory (LLNL); Energy Security: Janet Meier, Oak Ridge National Laboratory; Environmental Resilience: Sean Noble, Savannah River National Laboratory. LLNL’s Brandon Zimmerman, winner of the National Security research category, also took home the People’s Choice Award.
Featured Principal Investigators Videos
Five LLNL Principal Investigators introduce themselves and share some highlights of their recent LDRD project experience.